5 lessons from the Future Oil & Gas Digital Twin Conference
The recent Future Oil & Gas conference focused on the business, technical and cultural impact of Digital Twins and Artificial Intelligence (AI) on the Oil & Gas industry.
John Glen, Spartan Chief Financial Officer, was speaking and participating at the event and wanted to share the five key things we learned:
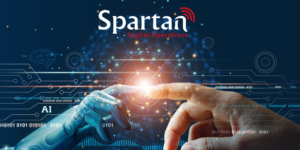
1. The Oil & Gas industry now has a clear definition of a Digital Twin
The technical definition that most experts agree upon is that a Digital Twin has three core elements:
Data
A repository of historical and live digital information bounded for the physical entity the twin represents. For example, to build a Digital Twin for Predictive Maintenance, you need historical telematics sensor data for the plant and equipment under surveillance. You should also include historical work orders and production loss events to provide operational context. This core data set can be augmented with other data sources such as weather feeds, P&ID Diagrams, Process Diagrams, and 3D models.
Models
The predictive algorithms that continuously analyse the Digital Twin and trigger warnings of predictive failure or process degradation in critical plant and equipment.
Visualisation
Several views into the Digital Twin that allow maintenance experts to explore, collaborate and validate model predictions. For example, the PROPHES Predictive Analytics tool provides time-series, card summary and heatmap views with an in-line comparison of trends to compare live performance against historical issues.
2. Put your people at the centre of the Tech
There is understandably confusion and concern around the introduction of Artificial Intelligence (AI) to support Oil & Gas Operations. But many attendees confirmed what we already knew – end-users are crucial to the success of any solution built on a Digital Twin.
For Predictive Maintenance, you need your equipment experts to work with data scientists to build accurate predictive models. And your Predictive Maintenance solution should present information as clearly as possible and support an integrated, cross-system workflow that accelerates the time from prediction to action.
John Glen, Spartan CFO, presented lessons Spartan has learned on building trust with users of Predictive Maintenance technology. Click here to see this short presentation and Q&A session.
3. Do not start without a clear value proposition
Anchor any project that uses Digital Twins and AI on a clear value proposition that the CFO can understand and measure.
The value proposition is clear and measurable for a Predictive Maintenance solution:
- How many additional barrels of production did we recover?
- How much unnecessary Operations and Maintenance activity did we remove?
- How many tonnes of CO2 emissions did we prevent?
One delegate mentioned that as much as 70% of maintenance is conducted at the wrong time. There is much value to be recovered by implementing the latest generation of cost-effective Asset Performance Management solutions.
4. Physics vs Maths? Don’t worry, be Hybrid
There was much debate around whether ‘white box’ predictive models based on the physics of plant and equipment were better than ‘black box’ predictive models based on mathematical algorithms generated by machine learning techniques.
The conclusion was that both approaches are valuable, and they should be used together in what is called a ‘hybrid’ Digital Twin.
5. The Tech is ready
There was a consensus that modern technology platforms like PROPHES are ready, if not slightly ahead, of the demand for AI applications such as Predictive Maintenance.
We now need to focus on the people, training and support of AI projects and use the Tech to provide a low-risk, low-cost platform to trial and, if successful, scale-up rollout at speed.
If you are interested in learning more about how our experts can help you implement Predictive Maintenance, send us an email or download our guide to Asset Performance Management for the Oil & Gas industry.
Tags: APM, Digital Twin, Oil & Gas